Overcoming Challenges in the Metalworking Industry with Industrial IoT
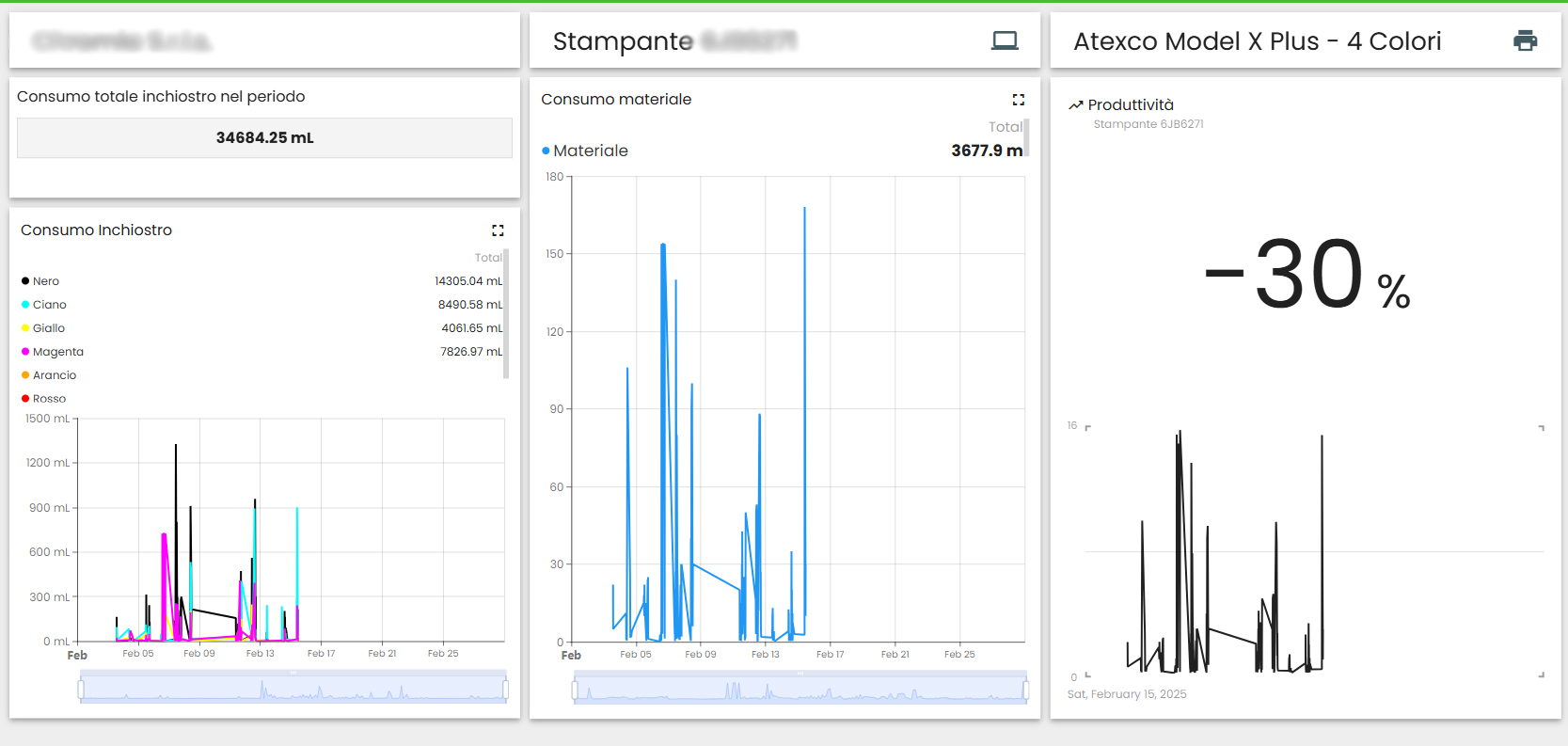
Figure 1. A custom Dashboard, created by Mative experts, to monitor the "Productivity" of a client's machines.
In the highly competitive landscape of the metalworking industry, small and medium-sized enterprises (SMEs) face a series of crucial challenges. Among these, monitoring and optimizing production emerges as one of the most pressing. Industrial IoT presents itself as a revolutionary solution, capable of radically transforming the way these companies operate and compete in the global market.
What is the situation of Metalworking SMEs?
SMEs in the metalworking sector are the beating heart of the Italian manufacturing industry. These companies face growing challenges: inflation, rising operating costs (especially energy), increasing competitiveness of foreign markets, and reduced operating margins (on average less than 10%). In this context, the need to reduce waste and optimize processes becomes crucial.
A modern and interconnected production monitoring system is essential to overcome these challenges and maintain competitiveness. The lack of real-time visibility into the status of machinery, production times, and overall plant efficiency represents a significant obstacle to optimizing production processes.
The adoption of industrial IoT solutions is the most effective response to these challenges. Advanced solutions such as the Mative Cloud and Mative Synapsis Industrial Edge platforms, specific for IoT and equipped with integrated AI, allow metalworking SMEs to overcome technological barriers, providing the necessary tools for a true digital revolution.
Identifying and reducing bottlenecks
One of the most significant advantages offered by an Industrial IoT system is the ability to quickly identify bottlenecks in the production process. Through data analysis, the Mative platform can highlight the stages that slow down the entire production cycle.
- Mative Cloud: remote monitoring, in the Cloud, accessible also through a mobile app.
- Mative Synapsis Industrial Edge: production chain monitoring, facilitating communication between departments, material consumption monitoring, integrated HMI.
- Mative Synapsis ML: the main core of Mative, a module based on Artificial Intelligence tools, available on all Mative platforms, integrates AI Agent, RAG, and Machine Learning;
- Mative Synapsis Analysis: ETL, Graphs, Smart Reports, and much more for a complete data analysis software suite;
These platforms, directly acting on the data collected from the machines, allow companies to intervene in a targeted manner, implementing specific solutions to increase overall efficiency.
Real-time monitoring: the key to efficiency
Real-time production monitoring is one of the main opportunities arising from the adoption of an industrial IoT system. The Mative Cloud platform allows the collection and analysis of data from every stage of the production process, providing crucial information on:
- Operational status of machinery
- Production times
- Efficiency of individual processing stages
- Energy consumption
- This immediate visibility allows managers to make informed and timely decisions, reducing downtime and optimizing resource allocation.
Predictive and conditional maintenance: how to avoid machine downtime
Predictive and conditional maintenance represents a qualitative leap compared to traditional reactive or preventive approaches.
Thanks to Industrial IoT systems, metalworking SMEs can constantly monitor the health of their machinery, identifying potential problems before they turn into failures. This proactive approach not only reduces unplanned machine downtime but also optimizes maintenance costs, extending the useful life of the equipment.
Optimization of energy consumption
In an era where sustainability and energy efficiency have become absolute priorities, industrial IoT offers valuable tools for monitoring and optimizing energy consumption.
The Mative platform allows detailed tracking of energy use for each machine and process, identifying areas of waste and opportunities for savings. This granular visibility enables companies to implement targeted energy efficiency strategies, reducing operating costs and environmental impact.
Complete visibility on production
Real-time monitoring is just the first step towards a true digital revolution in the metalworking industry. To transform this visibility into a concrete competitive advantage, companies need advanced tools capable of analyzing, interpreting, and acting on the collected data.
Designed to meet the specific challenges of metalworking SMEs, the Synapsis Industrial Edge and Mative Cloud Platforms integrate industrial IoT with powerful artificial intelligence algorithms, offering a complete digital ecosystem for production monitoring and process optimization.
The Mative Cloud Platform not only provides real-time data but also transforms it into valuable insights that support the informational needs of companies.
What are the key features of the Mative platforms?
The Mative platforms, Mative Cloud and Mative Synapsis Industrial Edge, offer a complete suite of features specifically designed for the needs of the metalworking industry:
-
Customizable Dashboards: Intuitive visualizations of the most relevant KPIs for industrial plant monitoring.
-
Advanced Analytics: data analysis tools to identify trends and improvement opportunities.
-
Intelligent Alerting: real-time notifications for anomalies or critical situations.
-
IoT Integration: simplified connection of new and legacy machinery to the digital platform.
-
Efficiency of metalworking SMEs with Mative: real cases
-
The adoption of industrial IoT through the Mative platforms is not just a matter of technology, but of business transformation. Metalworking SMEs that have embarked on this path have achieved significant results:
- Reduction of machine downtime by up to 30%
- Increase in production efficiency by 15-20%
- Optimization of energy consumption with savings of up to 25%
- Improvement in product quality and reduction of waste
- These results translate into a tangible competitive advantage, allowing companies to respond more quickly to market demands and offer superior quality products at lower costs.